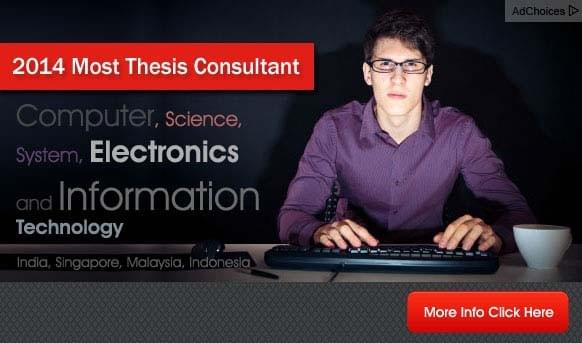
Program Algoritma Genetika Free Download Programs
The algorithm repeatedly modifies a population of individual solutions At each step, the genetic algorithm randomly selects individuals from the current population and uses them as parents to produce the children for the next generation. HERE
The evolution usually starts from a population of randomly generated individuals, and is an, with the population in each iteration called a generation.. Modifikasi Algoritma Genetika pada penelitian ini dilakukan berdasarkan temuan-temuan para peneliti sebelumnya tentang kelemahan Algoritma Genetika.. In each generation, the of every individual in the population is evaluated; the fitness is usually the value of the in the optimization problem being solved. https://priceless-morse-2a0345.netlify.app/Ssh-Generate-Public-Key-From-Private-Linux
Commonly, the algorithm terminates when either a maximum number of generations has been produced, or a satisfactory fitness level has been reached for the population.. A standard representation of each candidate solution is as an Arrays of other types and structures can be used in essentially the same way.. Contents Methodology [ ] Optimization problems [ ] In a genetic algorithm, a of (called individuals, creatures, or ) to an optimization problem is evolved toward better solutions. Click
A genetic algorithm (GA) is a method for solving both constrained and unconstrained optimization problems based on a natural selection process that mimics biological evolution.. The more fit individuals are selected from the current population, and each individual's genome is modified ( and possibly randomly mutated) to form a new generation.. Over successive generations, the population 'evolves' toward an optimal solution.. Variable length representations may also be used, but crossover implementation is more complex in this case. e10c415e6f Click
Once the genetic representation and the fitness function are defined, a GA proceeds to initialize a population of solutions and then to improve it through repetitive application of the mutation, crossover, inversion and selection operators.. The new generation of candidate solutions is then used in the next iteration of the.. Each candidate solution has a set of properties (its or ) which can be mutated and altered; traditionally, solutions are represented in binary as strings of 0s and 1s, but other encodings are also possible. HERE